Margrét Bjarnadóttir Directory Page
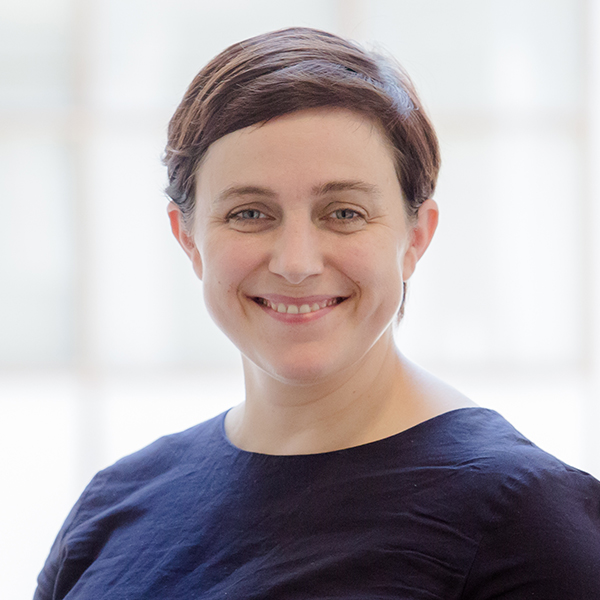
Margrét Bjarnadóttir
Associate Professor
Ph.D., MIT
Dr. Margrét Vilborg Bjarnadóttir is an Associate Professor of Management Science and Statistics in the DO&IT group. Dr. Margrét Bjarnadóttir graduated from MIT's Operations Research Center in 2008, defending her thesis titled “Data Driven Approach to Health Care, Application Using Claims Data”. Dr. Bjarnadóttir specializes in operations research methods using large scale data; her research centers around data driven decision making, combining optimization modeling with data analytics. Her work spans applications in health care, finance, people analytics and sports!
In health-care Dr. Bjarnadóttir specializes in decision modeling using EMR and claims data. Her recent papers include: “Aiding the Prescriber” which focuses on risk modeling for improved opioid prescriptions and “Predicting Colorectal Cancer Mortality” which utilizes EMR and Cancer Registry data to build decision support tools. Examples of other applications include drug surveillance design, practice patterns and patient targeting.
The focus of her People Analytics work is on algorithmic studies of demographic pay-gaps, including the best approaches to remedy the pay-gap problem taking into account both equity and the economics. In her recent paper “On a Firm's Optimal Response to Pressure for Gender Pay Equity” she highlights some of the unintended consequences of pay-gap legislation.
In finance, Dr. Bjarnadóttir studies complex networks. Her prior studies include analysis of nation-wide cross-ownership patterns and systemic risk. More recently she and her co-authors are studying the complex financial supply chains behind financial products.
Dr. Bjarnadóttir has consulted with both health care start-ups on risk modeling using health care data as well as governmental agencies such as a central bank on data-driven fraud detection algorithms.
News
Research
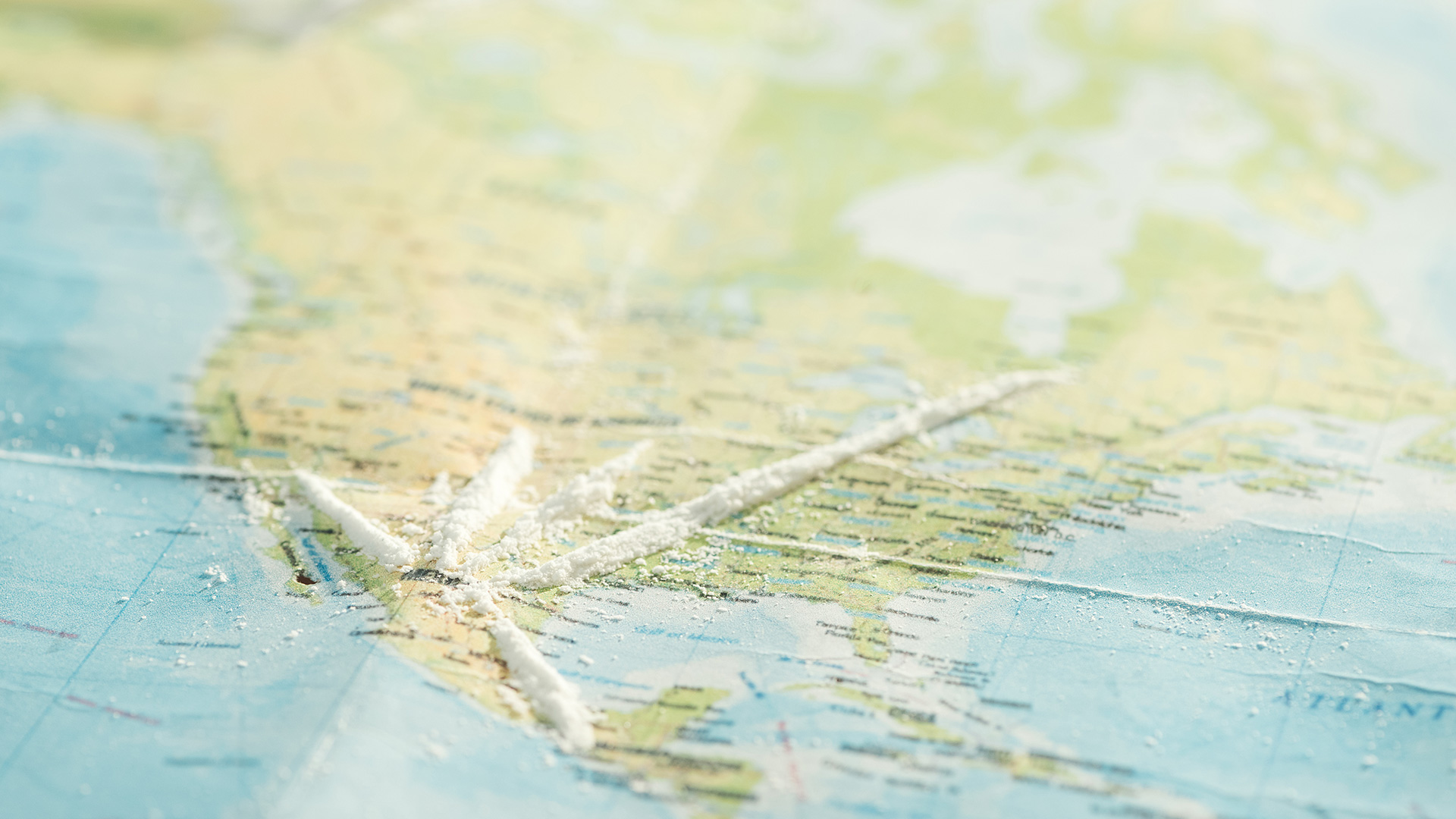


Insights

COVID-19 Widened Existing Inequities for Career Women. Here’s How We Get Back on Track.

New research may offer a way

New Research Shows How Telehealth Can Encourage Patient Self-Care
Academic Publications
Large language models and synthetic health data: progress and prospects
JAMIA Online, October 2024
There is growing interest in the application of machine learning models and advanced analytics to various healthcare processes and operations, including the generation of new clinical discoveries, development of high-quality predictions, and optimization of administrative processes. Machine learning models for prediction and classification rely on extensive and robust datasets, particularly for deep learning models common in health, creating an urgent need for large health datasets. Yet datasets can be insufficiently large due to the rapid evolution of diseases, such as coronavirus disease 2019 (COVID-19), rarity of disease, or the myriad obstacles to sharing and acquiring existing health data, including ethical, legal, political, economic, cultural, and technical barriers. Synthetic data provide a unique opportunity for health dataset expansion or creation by addressing privacy concerns and other barriers. In this paper, we review prior literature and discuss the landscape of machine learning models used for synthetic health data generation (SHDG), outlining challenges and limitations. We build on existing research on the state of the art in SHDG and prior broad explorations of the potential risks and opportunities for large language models (LLMs) in healthcare. We contribute to the literature with a focused assessment of LLMs for SHDG, including a review of early research in the area and recommendations for future research directions. Six promising research directions are identified for further investigation of LLMs for SHDG: evaluation metrics, LLM adoption, data efficiency, generalization, health equity, and regulatory challenges
Daniel Smolyak, Department of Computer Science, University of Maryland
Margret V. Bjarnadottir, Robert H. Smith School of Business, University of Maryland
Kenyon Crowley, Accenture Federal Services
Ritu Agarwal, Center for Digital Health and Artificial Intelligence, Carey Business School
Improved LISA Analysis for Zero-Heavy Crack Cocaine Seizure Data
INFORMS Journal of Data Science
Local Indicators of Spatial Association (LISA) analysis is a useful tool for analyzing and extracting meaningful insights from geographic data. It provides informative statistical analysis that highlights areas of high and low activity. However, LISA analysis methods may not be appropriate for zero-heavy data, as without the correct mathematical context the meaning of the patterns identified by the analysis may be distorted. We demonstrate these issues through statistical analysis and provide the appropriate context for interpreting LISA results for zero-heavy data. We then propose an improved LISA analysis method for spatial data with a majority of zero values. This work constitutes a possible path to a more appropriate understanding of the underlying spatial relationships. Applying our proposed methodology to crack cocaine seizure data in the U.S., we show how our improved methods identify different spatial patterns, which in our context could lead to different real-world law enforcement strategies. As LISA analysis is a popular statistical approach that supports policy analysis and design, and as zero-heavy data is common in these scenarios, we provide a framework that is tailored to zero-heavy contexts, improving interpretations and providing finer categorization of observed data, ultimately leading to better decisions in multiple fields where spatial data is foundational.
Eunseong Jang, The Robert H. Smith School of Business, University of Maryland
Margret Bjarnadottir, The Robert H. Smith School of Business, University of Maryland
Marcus Boyd, National Consortium for the Study of Terrorism and Responses to Terrorism, University of Maryland
S. Raghavan, The Robert H. Smith School of Business & Institute for Systems Research, University of Maryland