S. Raghu Raghavan Directory Page

S. Raghu Raghavan
Dean's Professor of Management Science and Operations Management
Ph.D. in Operations Research, Massachusetts Institute of Technology
Research Interests
Dr. Raghavan is passionate about using quantitative methods for better decision making. He enjoys teaching business analytics courses, and is a recipient of many teaching awards. These include (i) the INFORMS Prize for the Teaching of OR/MS Practice, (ii) the Legg-Mason Teaching Innovation award at the Smith School, and (iii) several Smith School Distinguished Teaching Awards. His research interests and activities cover a broad domain including---auction design, data mining, economics, information systems, computational marketing, logistics, health care policy and management, networks, optimization, and telecommunications. He has published on a wide variety of topics and numerous academic outlets such as Computers & Operations Research, Decision Sciences, Discrete Applied Mathematics, INFORMS Journal on Computing, Management Science, Networks, Operations Research, and Transportation Science. He holds two patents, and has won numerous awards for his work. These include (i) the Dantzig award for the best doctoral dissertation, (ii) the INFORMS Computing Society Prize (twice); once for innovative contributions to the field of data mining, and a second time for his contributions to public sector auction design, (iii) the Glover-Klingman Prize for the best paper in the journal Networks, (iv) the Management Science Strategic Innovation Prize by the European Operations Research Society, (v) the INFORMS Telecommunications Section Best Paper Award, (vi) 2nd Prize in the INFORMS Junior Faculty Paper Competition, (vii) Finalist for the European Operations Research Society Excellence in Practice Award, and (viii) Finalist for the Wagner Prize for Excellence in Operations Research Practice. Prior to joining the Smith School he led the Optimization Group at U S WEST Advanced Technologies.
News
Maryland Smith’s S.
Research
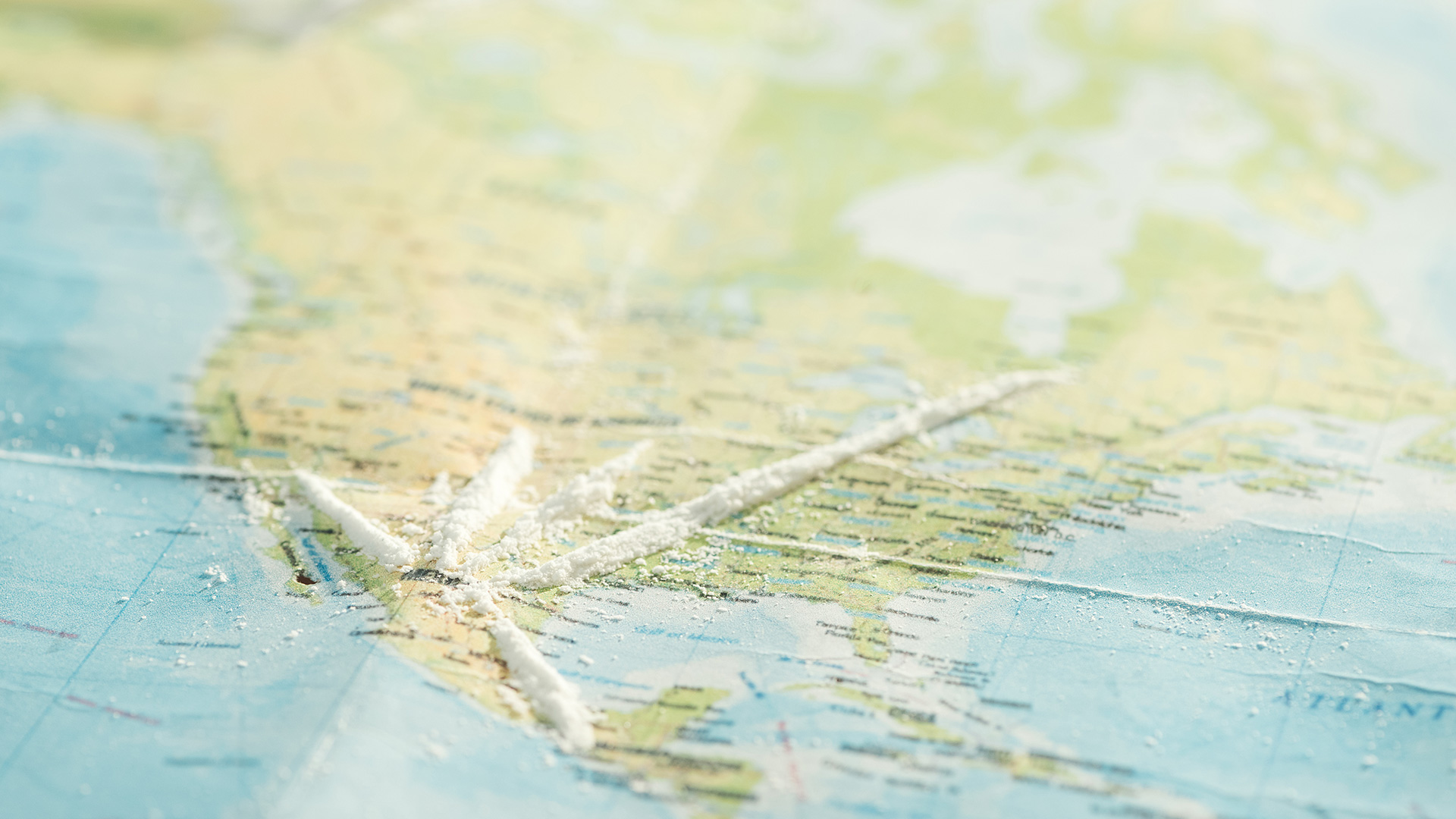
