Xiaojia Guo Directory Page
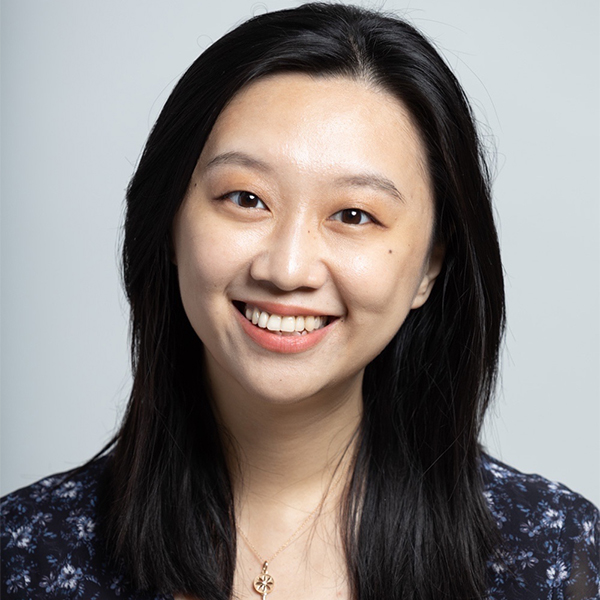
Xiaojia Guo
Assistant Professor
PhD, University College London
Xiaojia Guo is an Assistant Professor of Decision and Operations at the Robert H. Smith School of Business. Her research interests lie in predictive analytics and decision analysis. Specifically, her current research projects focus on probabilistic forecasting and combining predictions from experts or models, employing Bayesian statistics and machine learning techniques as primary methods. Xiaojia has won or been a finalist in several prestigious research awards, including the M&SOM Practice-based Research Competition, POMS-JD.com Best Data-Driven Research Paper Competition, INFORMS Aviation Applications Best Paper Award, INFORMS Decision Analysis Society’s Best Student Paper, and the Daniel H. Wagner Prize for Excellence in Operations Research Practice.
News
As courses convene for the new academic year, Maryland Smith is welcoming eight new scholars to its faculty.
Tejwansh (Tej)…
There will be a few new professors in the corridors of Van Munching Hall and in Maryland Smith’s online courses this academic year.…
Research

Academic Publications
Bayesian Ensembles of Exponentially Smoothed Life-Cycle Forecasts
Manufacturing and Servoce Operations Management
We study the problem of forecasting an entire demand distribution for a new product before and after its launch. Firms need accurate distributional forecasts of demand to make operational decisions about capacity, inventory and marketing expenditures. We introduce a unified, robust, and interpretable approach to producing these pre- and post-launch distributional forecasts. Our approach is inspired by Bayesian model averaging. Each candidate model in our ensemble is a life-cycle model fitted to the completed life cycle of a comparable product. A pre-launch forecast is an ensemble with equal weights on the candidate models’ forecasts, while a post-launch forecast is an ensemble with weights that evolve according to Bayesian updating. Our approach is part frequentist and part Bayesian, resulting in a novel form of regularization tailored to the demand forecasting challenge. We also introduce a new type of life-cycle or product diffusion model with states that can be updated using exponential smoothing. The trend in this model follows the density of an exponentially tilted Gompertz random variable. For post-launch forecasting, this model is attractive because it can adapt itself to the most recent changes in a product’s life cycle. We provide closed-form distributional forecasts from our model. In two empirical studies, we show that when the ensemble’s candidate models are all in our new type of exponential smoothing model, this version of the ensemble outperforms several leading approaches in both point and quantile forecasting. In a data-driven operations environment, our model can produce accurate fore- casts frequently and at scale. When quantile forecasts are needed, our model has the potential to provide meaningful economic benefits. In addition, our model’s interpretability should be attractive to managers who already use exponential smoothing and ensemble methods for other forecasting purposes.
Xiaojia Guo (Assistant professor, Robert H. Smith School of Business, UMD), Casey Lichtendahl (Google), Yael Grushka-Cockayne (Professor, Darden school of business, University of Virginia)