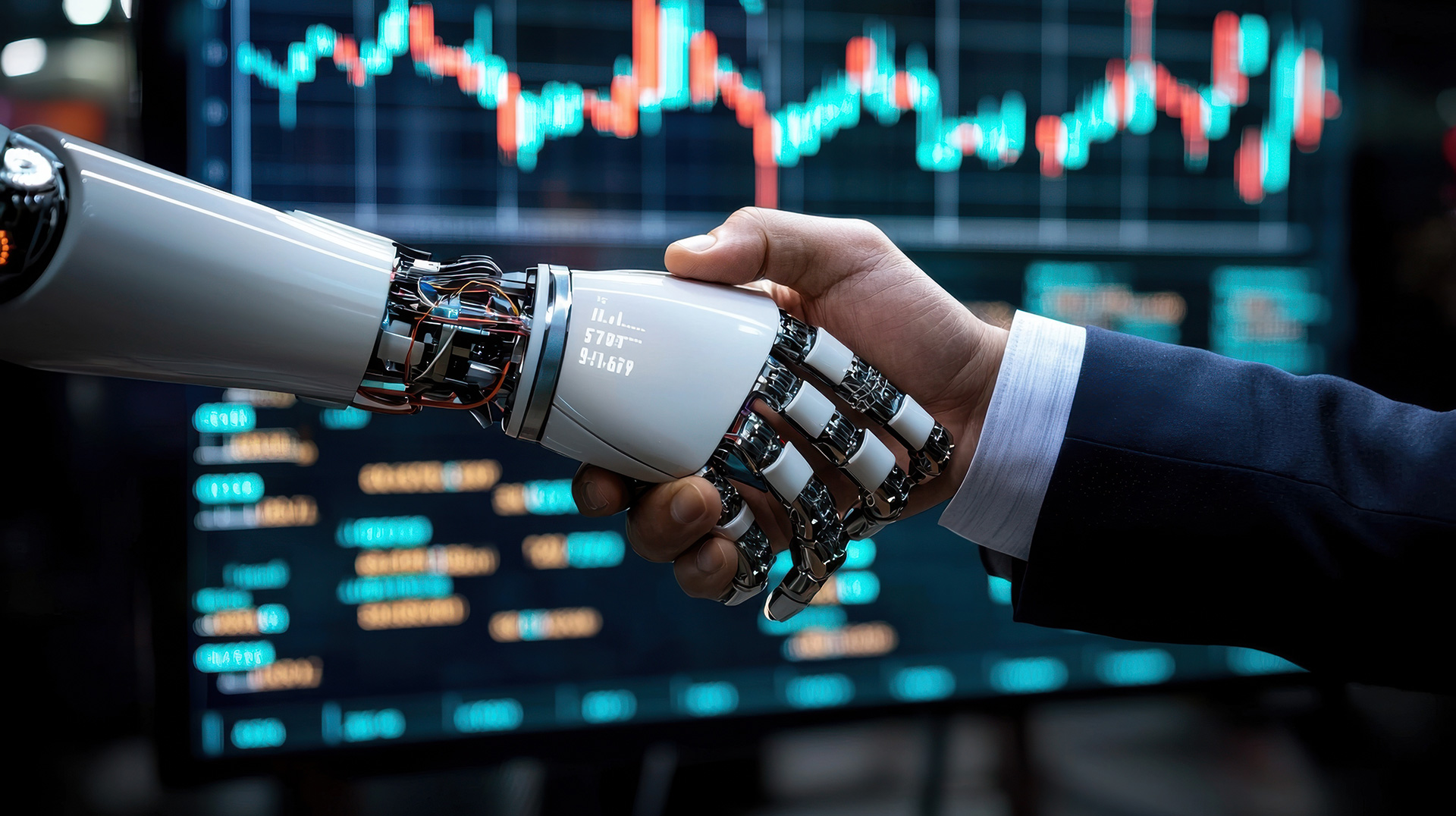
A lot has been made about the rise of artificial intelligence and if and when AI-powered machines will replace humans at work. For many jobs, the best outcomes will happen when humans team up with machines. That’s the case for stock analysts, finds new research from Sean Cao at the University of Maryland’s Robert H. Smith School of Business.
“The message here is the implication for the labor market,” says Cao. “We’re identifying the skills humans need to thrive in the age of AI – to be able to work with AI-powered machines instead of being replaced by them.”
Cao’s new research – with co-authors Wei Jiang (Emory University), Junbo Wang (Louisiana State University) and Baozhong Yang (Georgia State University) – is published in the October 2024 issue of the Journal of Financial Economics. They look at how skilled workers can tap into higher potential with help from AI technology, which is “presumably the primary goal for humans to design and develop AI in the first place,” they write.
The researchers look at how AI is changing the job for stock analysts, who are required to have both institutional knowledge and data analytics skills. More and more investors are heeding AI-powered recommendations to pick stocks and build their portfolios. But how do AI analysts stack up against human counterparts? That’s what Cao – director and co-founder of Smith’s AI Initiative for Capital Market Research – wanted to find out.
Cao and his co-authors built an AI-powered analyst of their own to predict 12-month stock returns. Their machine-learning tool used firm-level, industry-level and macroeconomic data, plus text analysis from sources including firms’ financial disclosures, news releases and social media. Cao deliberately excluded information from analyst forecasts – the researchers didn’t want their AI model to be swayed by human insights.
Cao then compared the AI’s predictions with human analysts’ forecasts made at the same time for the same stock.
No surprise: AI was clearly better at processing large volumes of data from public information, says Cao.
“We found that when firms have huge amounts of information – tax documents, press releases, etc. – machines did better at processes and synthesizing information,” Cao says. “But when a firm has a lot of intangible information – like a strong team, a lot of R&D, lots of knowledge capital – experienced human analysts are better at forecasting in those cases.”
The researchers’ AI analyst outperformed humans in 54.5% of the stock return predictions in the sample period of 2001 to 2018. When they controlled human analysts for biases, due to incentives or psychological traits, the humans outperformed AI in 46.5% of forecasts.
Cao was most interested in what gave humans the edge in the cases where they beat AI forecasts.
“Our paper focuses on identifying the things that humans are capable of doing but machines are not good at,” he says. “We found that humans can understand the institutional backgrounds of firms and industries well, but machines struggle with understanding that information. Because of that, a lot of Wall Street analysts will survive the age of AI.
The researchers also found that people affiliated with large brokerage houses were more likely to beat AI, thanks to a combination of their abilities and the research resources available to them. People were more likely to have the upper hand when a firm was operating in a rapidly changing competitive landscape, or subject to higher distress risk – like during the pandemic or the financial crisis – revealing AI’s limitations with unprecedented or unfamiliar situations.
When the researchers added the human analysts forecast to the AI model, the “man + machine” model beat the AI-only model. This was especially pronounced for the firms that the human analysts were better at predicting than the AI-only model.
People using AI will also drastically cut down on mistakes. The researchers’ man + machine model avoids about 90% of extreme errors made by human analysts and 40% of those made by AI-only.
“The biggest takeaway from the research is just how strong the outcome can be when people work with AI,” Cao says.
The research provides guidance on how humans can leverage their own strength and be better adapted in the age of AI, he says – a big focus for business schools, including the Smith School.
“We’re making changes in our curriculum to teach students how to work with AI,” says Cao. “A lot of recruiters told us they need students who understand AI. We need to prepare them for a world where humans work with machines.”
Read the research, “From Man vs. Machine to Man + Machine: The Art and AI of Stock Analyses,” in the Journal of Financial Economics.
Media Contact
Greg Muraski
Media Relations Manager
301-405-5283
301-892-0973 Mobile
gmuraski@umd.edu
Get Smith Brain Trust Delivered To Your Inbox Every Week
Business moves fast in the 21st century. Stay one step ahead with bite-sized business insights from the Smith School's world-class faculty.